So many possible utilizations
AI is already part of our life. Come back home and you could turn on your home system by voice control. A Spotify playlist recommends a list of new songs for a summer getaway. A modern electrical vehicle with no driver. These functionalities are the use of AI integrated with 5G connectivity, which gives us a more convenient lifestyle. Like other innovation technology, AI is created to help us solve something. In AI case, mostly make human programmer or user figure solution or pattern that is too difficult or time consuming for human. In the following article, I will show you further examples of existing AI applications and potential innovations.
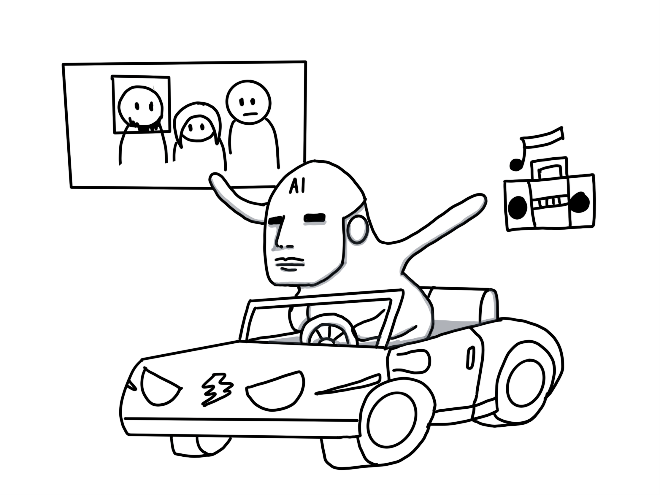
Case study: Autism Glass (Standford University)
A project that supports children with autism (a condition that patient has difficulty recognizing emotions, hence making social interaction tough). Autism Glass is a project by Standford University that incorporated psychiatry, behavior science, human-computer interaction, and AI. The project objective is to help the autistic child understand other people by integrating google glass, machine learning and real-time social cues to the user. Machine learning and AI analyze the emotion of people user encounters by collecting facial expressions from onward camera. The Autism glass then provides insight to the user. Data is also collected and shown on the smartphone of the patient, their parent, and medical professionals.
AI is also seen a lot in science fiction (Sci-fi). However, current AI or we called artificial narrow intelligence (ANI) and Sci-fi’s AI, which is called artificial general intelligence (AGI) are quite different. The latter understand and react to a real-world situation like what we found in movies and books. Mainstream media often write exaggerate AI innovation. Mostly compare it to pop culture understanding of the topic. However, ANI that we currently have a much less refined and still need along way to be fully automated with human programmer assist.
Brain power of a worm
As written earlier, AI is already integrated into our daily life, in smartphones, websites and ongoing research that tackle a lot of data. With the improvement of the technologies, the rules-based program will be replaced with AI. Instead of learning only spreadsheets, the future workforce also needs to understand how machine learning works or data science in general (see further detail in the data world). Once given a proper dataset, AI will give us (hopefully) the correct solution we want. But there is a catch on all this. Janelle Shane [1] mentioned earlier in her book that AI is not smart, and it depends on the given dataset and the problem we want it to solve. Sometimes it learns mistakes from flawed data and other times the problem is too hard for AI to crack. In general, programs design AI to solve the problem for us the way humans solve the problem, it will always look for shortcuts, and most of the time it doesn’t understand the problem the programmer wants it to solve. (And AI has the brainpower of worm).
Programmers and developers must make sure AI is used in practical and suitable situations. A miscalculation or misunderstanding of the program could bring the user a great loss. A good example is a self-driving car that still needs the human driver to sit and take over control if AI ever lost its ability to judge correctly.

Janelle [1] wrote in her book the characteristic of artificial intelligence that people should understand while let it handle any tasks. What AI does is not a recommendation, but a forecast from data set that is given to the machine learning algorithms. These points still need human to operate the AI and make sure the machine perform its tasks correctly.
1. AI has really limited brain power; it could work well with very narrow and tightly defined problem. If there are many tasks or requirement, the program probably build a neural network of AI with many different AI perform its singular tasks. If the problem is too broad or need context to solve, human still required in the process.
2. A dataset that trained AI is also another problem. AI could only perform its task based on given dataset for the training. If it is given a dataset with irrelevant information, the AI will be confused, which in turn provide a wrong solution. The type of data is also important. If the machine is given or trained with photo as input, the AI will provide output as a photo or related to the photo. Let’s say there a picture of a man and woman in a café in Paris, the AI could recognize ‘man’, ‘woman’, café and landscape of may be the Eifel tower. But it could not predict whether this is a date, a business meets up or what could be any other related information of that photo because the AI could not provide contextual within that photo. Unless there is human, or another AI supports the analysis.
3. AI is bad with memory. It could not do a long-term strategy and it forget previous task easily, unless it is programmed to remember specific important information. Any task of problem that requires recalling information from previous tasks or information still need human editor.
4. AI copies human bias. With given dataset, AI could give decision based on human bias based on original dataset, despite programmer intention not to. Racism and sexism could potential be integrated into AI. For example, with a given data of hired employee might make AI select people of color less or choose man before woman.